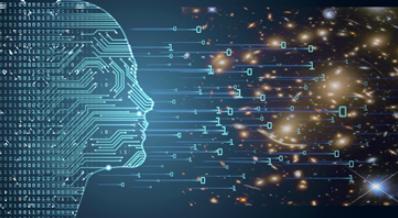
Data science
Phd Thesis
Site: Milano
Durata
3 years
Tutors
Stefano Andreon (INAF-OAB), Marco Landoni (INAF-OAB) e Alberto Trombetta (UniInsubria)
Contact
stefano.andreon AT inaf.it
marco.landoni AT inaf.it
Description
Computation of Bayesian posterior probabilities, often performed via Monte Carlo Markov Chain sampling, is perhaps the most common method used by astronomers and physicists to estimate cosmological or astrophysical parameters. It is now becoming increasingly computationally demanding with the incoming avalanche of data coming from Euclid, SKA, Lofar, and Rubin telescopes, all of which are prime INAF flagship projects. New advanced methods to improve the efficiency of the posterior sampling exist, for example just-in-time compilation, automatic differentiation, hamiltonian montecarlo, advanced gradient-based sampling techniques, and normalizing flow.
This thesis aims to compare the performances of the available methods, to combine them, and to make available their optimal combination to the astrophysical community.
Our goal is to offer a solution that does need to overhaul the code to the hardware/software environment, but can be deployed out of the box with minimal integration effort.
This project is integrated in the Milan Area Data Science group, including Marco Landoni (Brera), Alberto Trombetta (UniInsubria), Fabio Castagna (Brera & Insubria).
[Image credit: University of Ohio]